Welcome to Computational Statistics and Machine Learning
The members of the Computational Statistics and Machine Learning Group (OxCSML) have research interests spanning Statistical Machine Learning, Monte Carlo Methods and Computational Statistics, and Applied Statistics.
Research in Statistical Machine Learning spans Bayesian probabilistic and optimization based learning of graphical models, nonparametric models and deep neural networks, and complements research in Monte Carlo methods for related classes of problems.
Research in Applied Statistics motivates the more theoretical work in this group and some staff focus on developing statistical methodology ‘on demand’ in a wide range of application domains.
Join us for doctoral study
The group carries out a broad range of computational biology research including Genetics, Genomics and Epidemiology. The research is both theoretical and applied, generating both new methods and genetic and epidemiological insights as well as computational tools and software. In Statistical Genetics we work to identify how mutations drive variability among people in health and disease risk, to understand the history of our and other species, and to understand the forces that have shaped evolution across the tree of life, whilst in Epidemiology we work to gain robust insights into the transmission and control of outbreaks, epidemics and pandemics of infectious diseases including COVID-19, Ebola, H1N1 influenza, MERS, rabies, dengue and Zika.
Within the University of Oxford, we have close links to the Wellcome Centre for Human Genetics, the Pandemic Sciences Institute and the Big Data Institute. Members of the group have played central roles in some of the most important international collaborative projects in human genetics such as the HapMap Project, the Wellcome Trust Case-Control Consortium, the 1000 Genomes Project, the People of the British Isles Project, the Haplotype Reference Consortium, UK Biobank and the 100,000 Genomes Project. Others have worked collaboratively with the World Health Organization.
Join us for doctoral study
Our research group is truly collaborative. Most epidemiology students are jointly supervised by someone based elsewhere (including other University of Oxford departments such as Biology, the Nuffield Department of Medicine and the Mathematical Institute) or other organizations (including the World Health Organization, the Zoological Society London, the UKHSA, the University of Liverpool and Liverpool School of Hygiene and Tropical Medicine). We currently have around 20 research students.
Take a look at our research, and if you're interested, get in contact.
Working in a group with such a wide range of interconnected research, from examining the social implications of public health policy to proving mathematical properties of epidemiological models, provides a great opportunity for learning and collaboration
Matthew Penn, DPhil Student
Welcome to the Oxford Protein Informatics Group (OPIG)
Based in the Department of Statistics, OPIG is a dynamic, collaborative and interdisciplinary group that investigates antibodies, small molecules, and proteins. Together with a number of academic and industrial collaborators, OPIG blends experimental data and cutting-edge computational method development to gain valuable insights in these fields. The myriad of approaches OPIG employs includes deep learning, generative models, and computational statistics, as well as more traditional physics-based approaches. OPIG has a long history of collaborating closely with industry on key challenges in small molecule drug and biologics discovery.
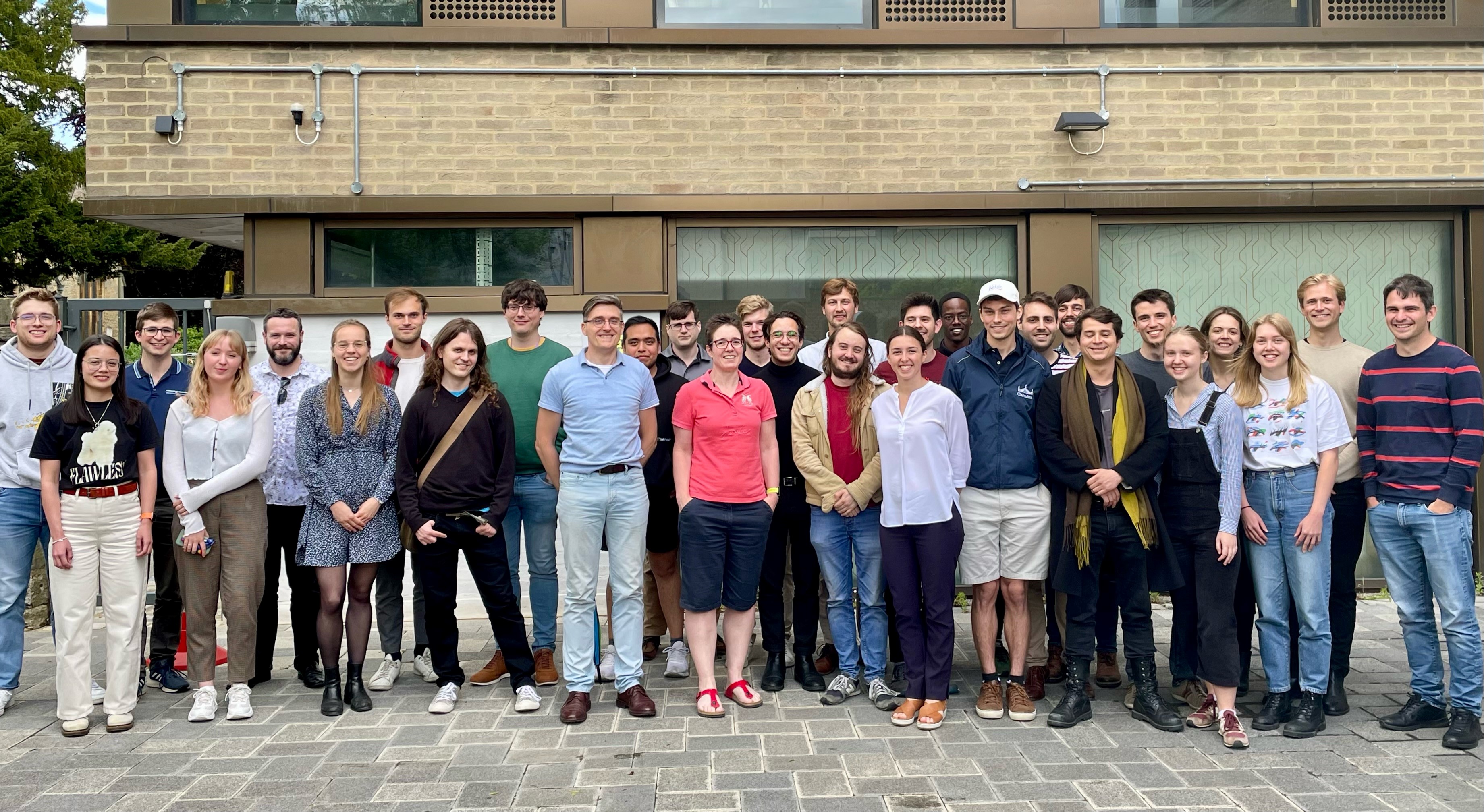
Immunoinformatics
The immunoinformatics subgroup develops machine learning and bioinformatics methods for antibody structure prediction, optimisation and design, as well as infectious disease research.
Protein Structure
The protein structure subgroup develops methods to understand protein folding and conformation, using theoretical, computational, and experimental approaches. In collaboration with Diamond Light Source, we also develop advanced methods for X-ray crystallography.
Small Molecules
The small molecules subgroup focuses on the development and application of computational methods to small molecule drug discovery. These include protein-ligand docking, scoring function development and binding affinity prediction, virtual screening, fragment-based drug discovery, de novo design, and lead optimization.
Welcome to Computational Biology and Bioinformatics
This research group develops computational and statistical methods for the analysis of such data, with particular emphasis on methods for biological networks and biological sequences. The analysis of biological data such as DNA sequences, gene expression arrays and single cell data can reveal new insights into intracellular mechanisms as well as evolutionary processes.
Bioinformatics and Computational Biology do not have formal definitions that anybody has to adhere to, but would typically be understood as follows:
Bioinformatics is today extremely diverse and can include data sources from multiple levels: Sequence Data remains a very dominating form of data, but are crucially supplemented by structural information (small molecules, macromolecules such as protein, RNA and more), expression levels of genes, concentrations of molecules in cells and tissues, and phenotypes. Such data can be analysed by simple stochastic models or lately by machine learning techniques that can use (and needs) huge amounts of very heterogenous data.
Computational Biology has a much wider domain and include large topics such as Computational Neuroscience, Computational Embryology, Whole Cell Modelling, Biosphere Modelling and more. Computational Biology also include a larger suite of techniques such a Dynamical Systems, Partial Differential Equations, Physical Chemistry, Dynamics on Networks and more.
The recent twin crises of the COVID-19 pandemic and climate change has highlighted the importance of Bioinformatics and Computational Biology. Understanding the pandemic spread has relied heavily on epidemiological models and sequence data, while structural information has been crucial in finding weak points of the SARS CoV-2 virion and its replication. Understanding the detailed consequences of climate change cannot be done without modelling of the biological components (e.g. the physiology of individuals, populations, species, and ecosystems) of the biosphere under slightly changed circumstances.
These computational approaches to the biosciences will continue for decades and could eventually achieve very detailed simulations of biological systems. The Department is very enthusiastic about this development and determined to be a key player contributing to these ambitious endeavours.
Breadcrumb
Professorial Research Fellow, Deputy Head of Department
Fellow of the British Academy
Fellow of the International Association for Applied Econometrics
Co-Editor of the Journal of Applied Econometrics
Associate Editor of the Journal of the American Statistical Association
Recent publication:
The robust F-statistic as a test for weak instruments , Journal of Econometrics
Stata code for command gfweakivtest
Biographical Sketch
- 2005-2019 Professor of Econometrics, University of Bristol
- 2002-2005 Co-Director, Centre for Microdata Methods and Practice
- 1996-2005 Senior Researcher, Institute for Fiscal Studies
- 1994-1996 ERC Post-Doc, University College London
- 1992-1994 Visiting Assistant Professor, Australian National University
Research Interests
- Causal inference
- Instrumental variables estimation, weak/invalid instruments
- Mendelian randomisation
Publications
Welcome to Econometrics and Population Statistics
The Econometrics and Population Statistics group develops and deploys statistical and mathematical methods to resolve questions that arise from the quantitative study of populations, markets and interventions. These include the exploration of social, economic, financial, ecological, and population-level health phenomena.
Algorithms and data science
The development and mathematical/statistical analysis of algorithms that extract information from high-dimensional noisy data sets, network time series, and certain computationally-hard inverse problems on large graphs. Particular areas of focus include the statistical analysis of big financial data, statistical arbitrage, market microstructure, limit order books, synthetic data generation, as well as nonlinear dimensionality reduction techniques for high-dimensional time series data. (Lead: Mihai Cucuringu)
Causal Inference
Causal inference, in particular the identification and estimation of causal effects in situations where standard estimation techniques are invalid due to the presence of unobserved confounders. Research focuses on Instrumental Variables estimation, in particular testing for underidentification, weak instruments and the performance of weak instruments robust inference, and selection of valid instruments, incorporating machine learning techniques. These methods are applied across many fields of study, including biostatistics, epidemiology, where Mendelian Randomisation studies use genetic markers as instrumental variables for modifiable phenotypes, social sciences and asset pricing models in finance. (Lead: Frank Windmeijer)
Population Statistics
The group is actively involved in exploring statistical and mathematical problems that arise from the study of biological populations. Much of this work is devoted in particular to questions of biological ageing, including problems of mathematical evolutionary theory, mathematical ecology, methods for longitudinal social and medical data, and survival analysis. This work implicates a broad range of technical areas, from stochastic dynamical systems through Bayesian computation to kernel methods and deep learning, and applies these tools to relevant data that will help to illuminate fundamental issues about life history in humans and other organisms. (Lead: David Steinsaltz)
Join us for doctoral study
Welcome to Oxford Probability
Oxford has a large and thriving community of researchers in probability, spanning the Department of Statistics and the Mathematical Institute. Within the Department of Statistics, our research interests include interacting particle systems, random trees and branching processes, random graphs and networks, percolation, mathematical population genetics, stochastic analysis and Stein’s method.
We work closely with the Stochastic Analysis group in the Mathematical Institute.
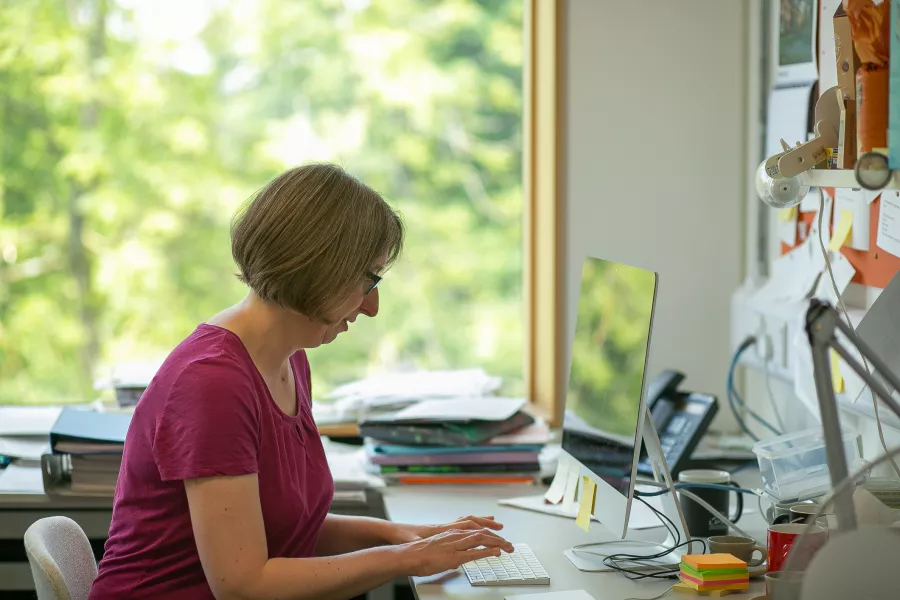
Our People
The Probability group includes 7 permanent faculty, around 15 doctoral students and a few postdocs .
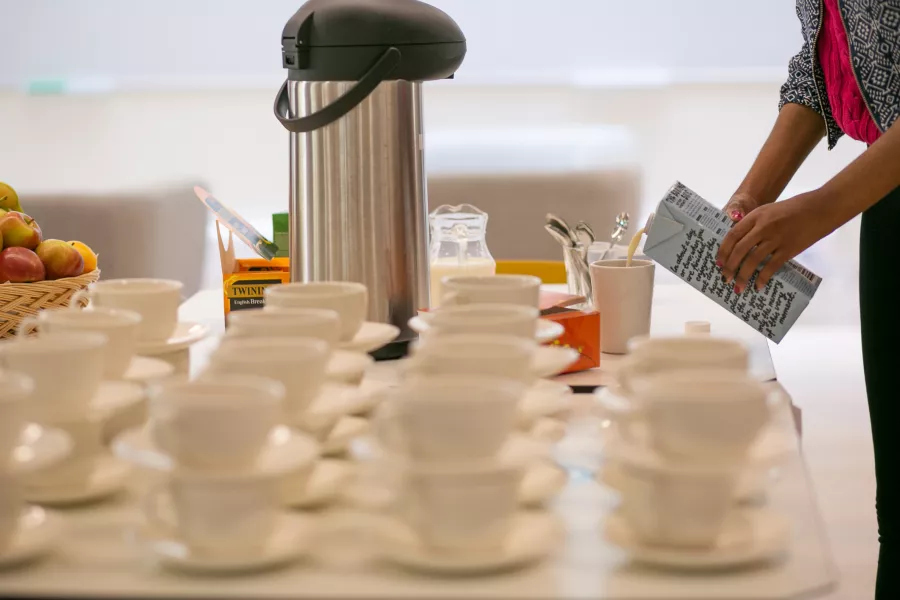
Events
We organise a weekly Probability Seminar. We are also involved in the online Oxford Discrete Mathematics and Probability Seminar, which currently takes place a couple of times a term, and Oxford Research on Probability and Machine Learning which runs termly seminars.
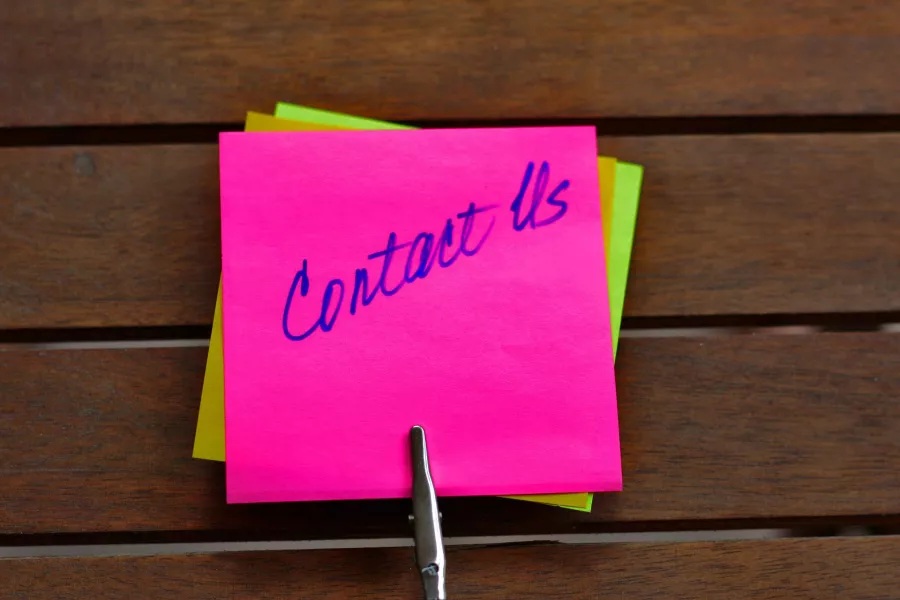
Keep in Touch
You can stay informed about what we do by subscribing to the Oxford Probability Mailing list.
Join us for doctoral study
We take both standard DPhil students and DPhil students through the EPSRC CDT in the Mathematics of Random Systems which is run jointly between the Mathematical Institute, the Department of Statistics and the Department of Mathematics at Imperial College.
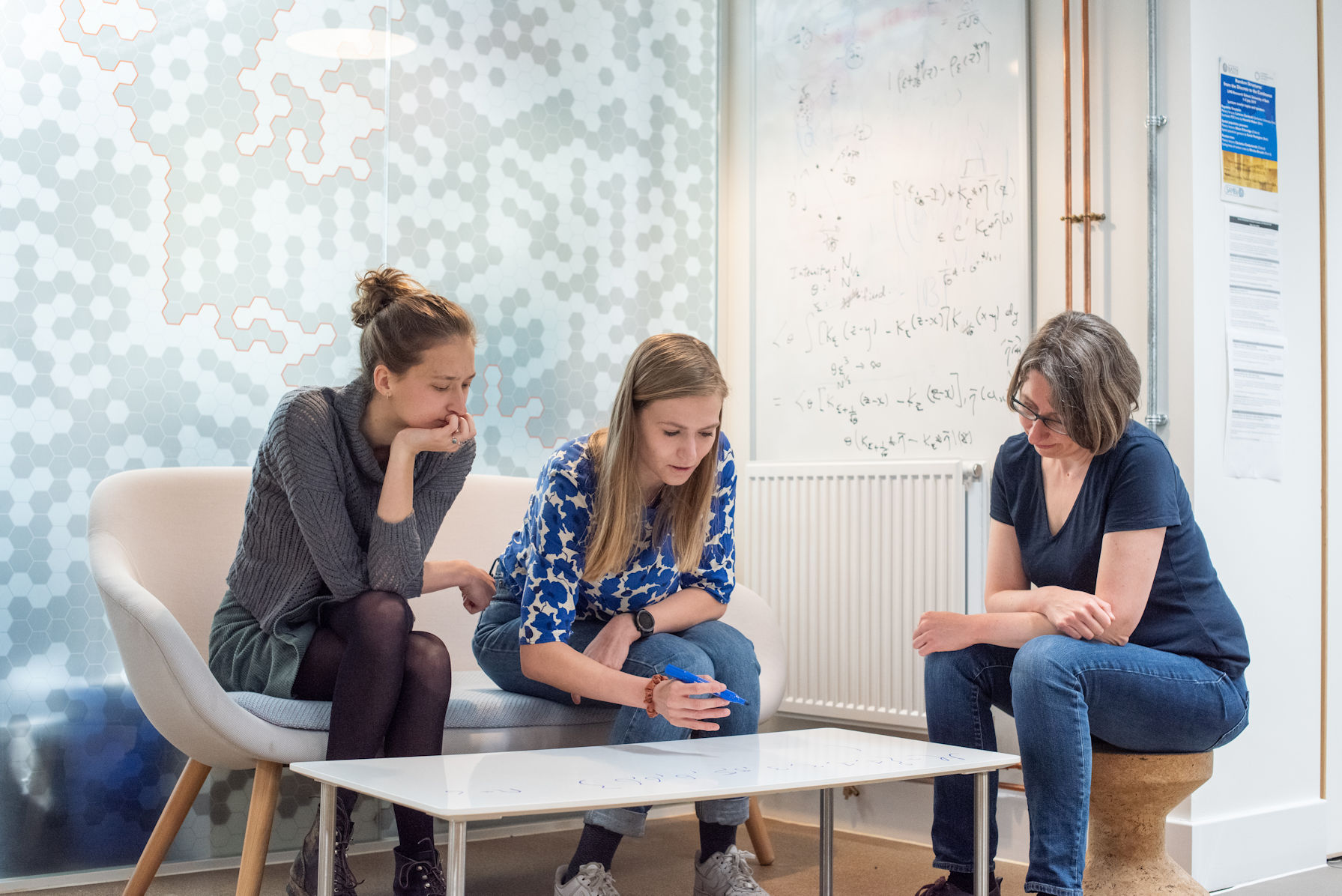