Please also see our full list of publications.
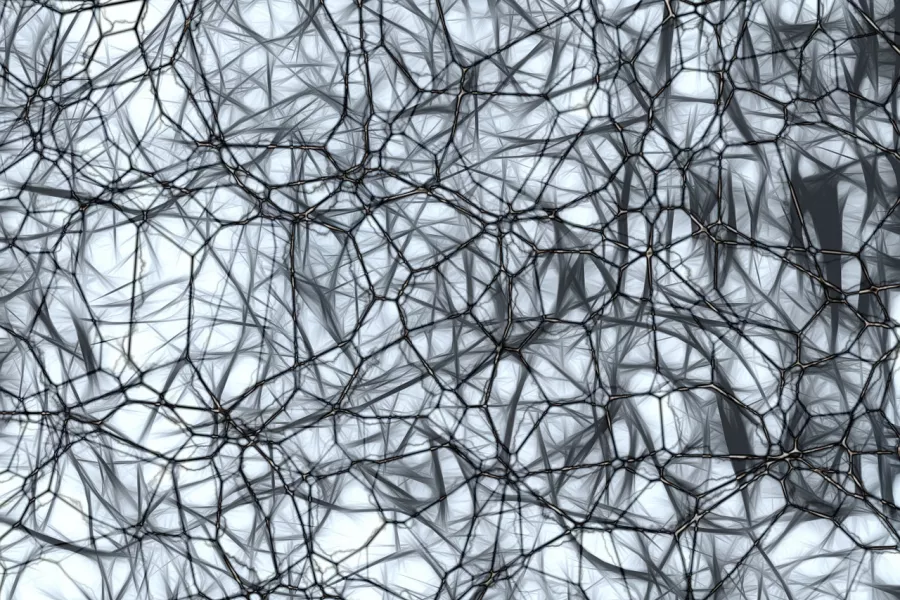
Neuroscience
Modelling in neuroscience has had huge successes in recent years and decades. Artificial neural networks can do more and more of what was once viewed only possible by biological brains: memory, cognition and language processing to name a few. However, this is easier than demonstrating these abilities in a way that biological networks do and full scale modelling of even small biological neural networks such as C. elegans (302 neurons), the fruit fly (hundreds of thousands of neurons) and mice (hundreds of millions of neurons) could be well into the future.
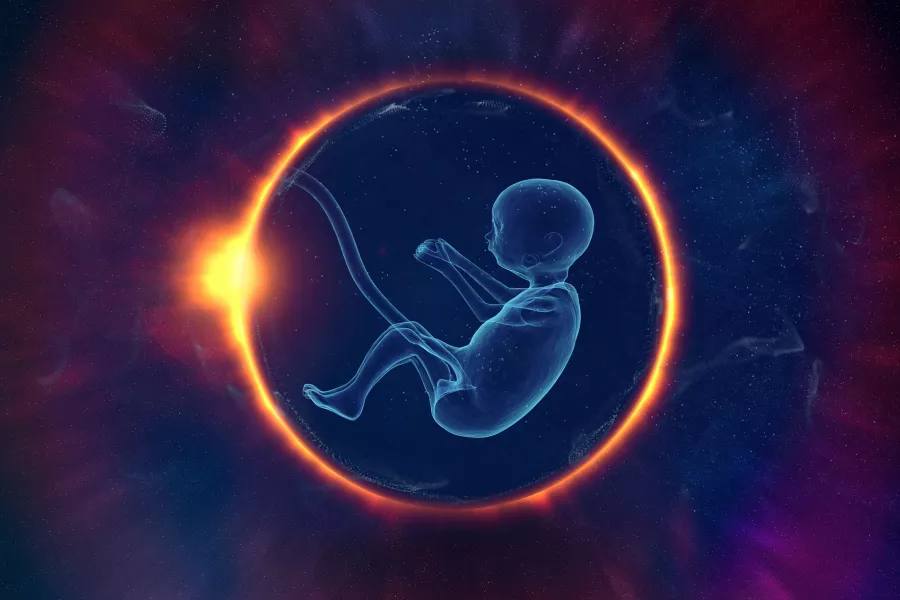
Embryology
Embryology is the development of a complete individual from a single cell with its genome. The phenotype of an organism are all the characteristics needed to characterise that organism, for example form, physiology, or behaviour. Going from genome to phenotype is one of the few problems that could be called the Holy Grail of Biology. And within phenotype, form has historically been central. Attempts are being made to predict facial features from the genome only recently, but solving this in general belongs to the future.
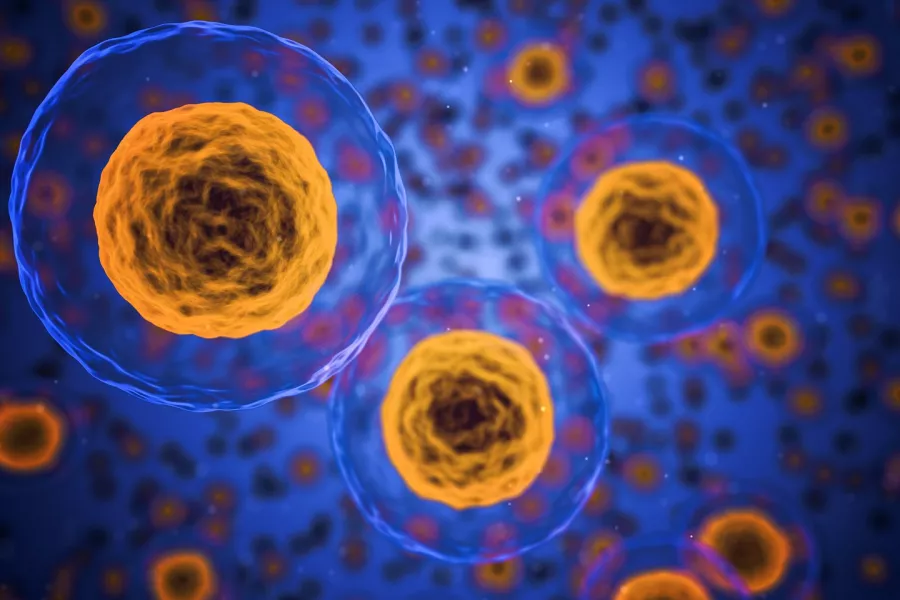
Whole Cell Modelling
Much progress has been made in modelling the components of a cell and their dynamics: small molecules, macromolecules, assemblies, membranes, chromosomes and networks. The smaller the easier – both to get the necessary data to parametrise the models and computationally to run the models. Going from the components to the complete cell is a non-trivial – there are 8-12 orders of magnitude difference in size between a protein and a complete cell. It is impossible to know how even small errors in prediction will influence a whole cell model, so this task is only completed when a virtual cell can faithfully mimic the behaviour of complete cells. The first attempts were in the 1990s and it might well be another decade before the goal is achieved.

Analysis of Biological Networks
Networks are increasingly used to represent complex dependencies between sub-cellular entities, such as protein interaction networks and gene co-expression networks. With increasing amount of such network data becoming available, many statistical questions arise. The standard framework of statistical inference is that of independent and identically distributed observations, but in data from networks this paradigm is clearly broken - it is the complex dependence between observations which gives rise to a network representation in the first place. Hence a key statistical question is how to incorporate the network dependence in statistical inference.
In particular, protein interaction networks or gene co-expression networks from different species may contain an evolutionary signal. Deriving methods for finding such signals, through network comparison statistics, has been a focus of attention in our group.
Some understanding the theoretical behaviour of such comparison statistics, which are often based on subgraph counts, can be gained by using different probabilistic models for networks. This research thus reaches into probability theory.
From an applied angle, network representations can reveal possible drug targets or possible interventions for enhancing nitrogen uptake in a legume. Members of our group have collaborated for example with Philip Poole from the Department of Zoology as well as with industrial partners such as Novo Nordisk and e-Therapeutics to tackle these questions.

Modelling of host-parasite systems
The development of theoretical and applied computational biology has increased the importance of mathematical modelling and simulation, paralleling the demand for more quantitative insight into challenging biological and epidemiological problems. Broadly, mathematical models in computational biology can be categorised as population-based models (PBMs), individual-based models (IBMs) or hybrid models (which leverage the relative advantages of PBMs and IBMs). PBMs (e.g., continuous-time, discrete-time, and stochastic models) can predict and understand population dynamics, although they frequently ignore the system's underlying biological realism due to mathematical generalities. IBMs circumvent the limitations of PBMs by allowing for a high level of individual and interaction complexity via in-silico modelling, but they also are criticised for lacking a more formal framework and analytical procedures compared to PBMs. Fitting complex mathematical models via likelihood-dependent estimation methods (e.g., maximum likelihood and classical Bayesian approaches) can pose significant challenges, especially when the likelihood function is either computationally expensive to evaluate or mathematically intractable. Consequently, a class of likelihood-free parameter estimation techniques known as approximate Bayesian computation (ABC) can overcome such estimation difficulties in the classical Bayesian setting.